You do not need to falsify your results if you are happy to draw conclusions contrary to the outcome of your data analysis.
Keith S. Taber
Li and colleagues claim that their innovation is successful in improving teaching quality and student learning: but their own data analaysis does not support this.
I recently read a research study to evaluate a teaching innovation where the authors
- presented their results,
- reported the statistical test they had used to analyse their results,
- acknowledged that the outcome of their experiment was negative (not statistically significant), then
- stated their findings as having obtained a positive outcome, and
- concluded their paper by arguing they had demonstrated their teaching innovation was effective.
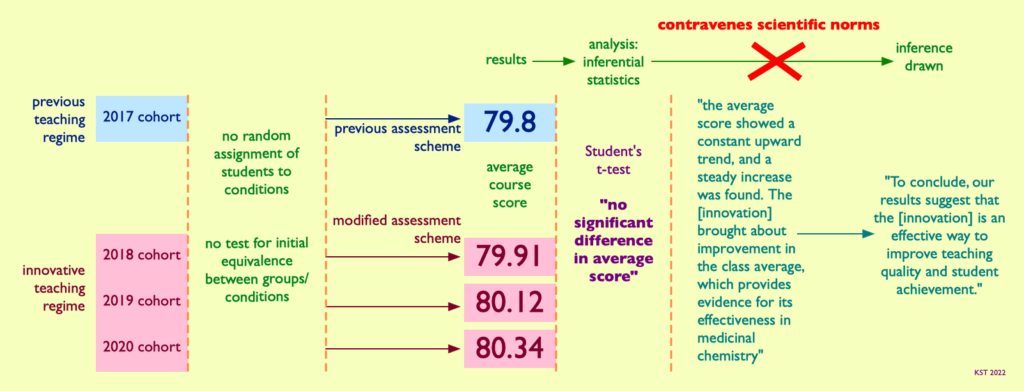
(Magnified portions of this scheme are presented below)
And this was not in a paper in one of those predatory journals that I have criticised so often here – this was a study in a well regarded journal published by a learned scientific society!
The legal analogy
I have suggested (Taber, 2013) that writing up research can be understood in terms of a number of metaphoric roles: researchers need to
- tell the story of their research;
- teach readers about the unfamiliar aspects of their work;
- make a case for the knowledge claims they make.
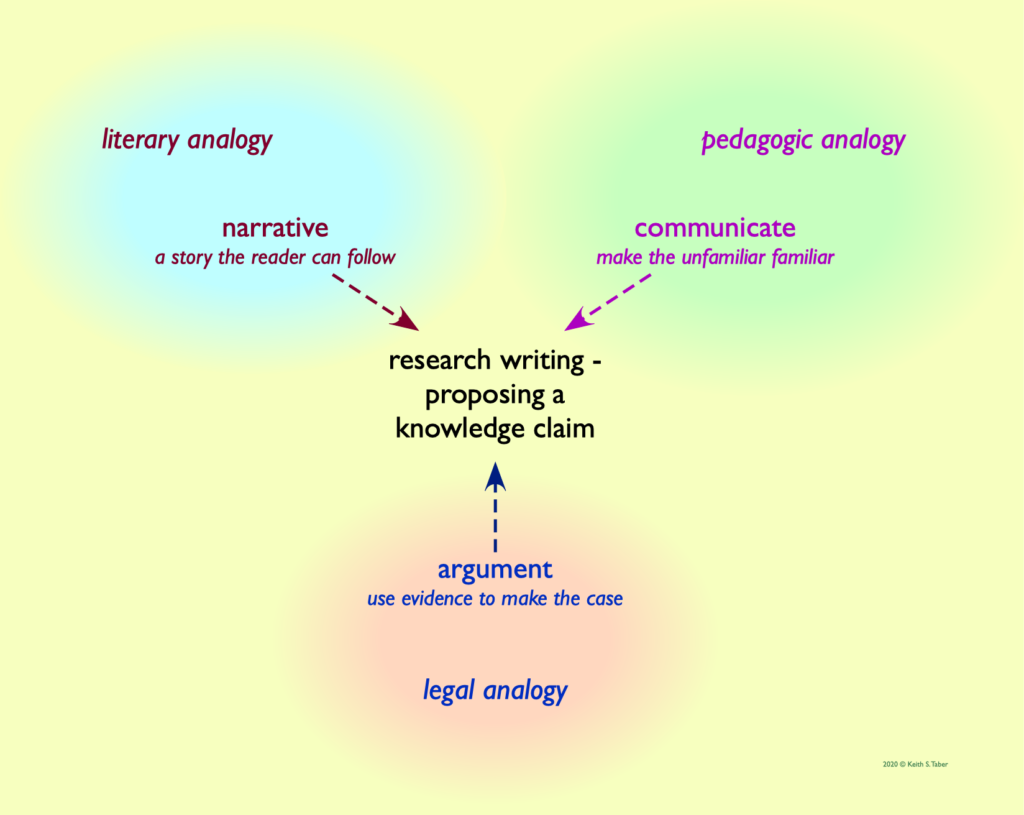
All three aspects are important in making a paper accessible and useful to readers, but arguably the most important aspect is the 'legal' analogy: a research paper is an argument to make a claim for new public knowledge. A paper that does not make its case does not add anything of substance to the literature.
Imagine a criminal case where the prosecution seeks to make its argument at a pre-trial hearing:
"The police found fingerprints and D.N.A. evidence at the scene, which they believe were from the accused."
"Were these traces sent for forensic analysis?"
"Of course. The laboratory undertook the standard tests to identify who left these traces."
"And what did these analyses reveal?"
"Well according to the current standards that are widely accepted in the field, the laboratory was unable to find a definite match between the material collected at the scene, and fingerprints and a D.N.A. sample provided by the defendant."
"And what did the police conclude from these findings?"
"The police concluded that the fingerprints and D.N.A. evidence show that the accused was at the scene of the crime."
It seems unlikely that such a scenario has ever played out, at least in any democratic country where there is an independent judiciary, as the prosecution would be open to ridicule and it is quite likely the judge would have some comments about wasting court time. What would seem even more remarkable, however, would be if the judge decided on the basis of this presentation that there was a prima facie case to answer that should proceed to a full jury trial.
Yet in educational research, it seems parallel logic can be persuasive enough to get a paper published in a good peer-reviewed journal.
Testing an educational innovation
The paper was entitled 'Implementation of the Student-Centered Team-Based Learning Teaching Method in a Medicinal Chemistry Curriculum' (Li, Ouyang, Xu & Zhang, 2022), and it was published in the Journal of Chemical Education. 'J.Chem.Ed.' is a well-established, highly respected periodical that takes peer review seriously. It is published by a learned scientific society – the American Chemical Society.
That a study published in such a prestige outlet should have such a serious and obvious flaw is worrying. Of course, no matter how good editorial and peer review standards are, it is inevitable that sometimes work with serious flaws will get published, and it is easy to pick out the odd problematic paper and ignore the vast majority of quality work being published. But, I did think this was a blatant problem that should have been spotted.
Indeed, because I have a lot of respect for the Journal of Chemical Education I decided not to blog about it ("but that is what you are doing…?"; yes, but stick with me) and to take time to write a detailed letter to the journal setting out the problem in the hope this would be acknowledged and the published paper would not stand unchallenged in the literature. The journal declined to publish my letter although the referees seemed to generally accept the critique. This suggests to me that this was not just an isolated case of something slipping through – but a failure to appreciate the need for robust scientific standards in publishing educational research.
Read the letter submitted to the Journal of Chemical Education
A flawed paper does not imply worthless research
I am certainly not suggesting that there is no merit in Li, Ouyang, Xu and Zhang's work. Nor am I arguing that their work was not worth publishing in the journal. My argument is that Li and colleague's paper draws an invalid conclusion, and makes misleading statements inconsistent with the research data presented, and that it should not have been published in this form. These problems are pretty obvious, and should (I felt) have been spotted in peer review. The authors should have been asked to address these issues, and follow normal scientific standards and norms such that their conclusions follow from, rather than contradict, their results.
That is my take. Please read my reasoning below (and the original study if you have access to J.Chem.Ed.) and make up your own mind.
Li, Ouyang, Xu and Zhang report an innovation in a university course. They consider this to have been a successful innovation, and it may well have great merits. The core problem is that Li and colleagues claim that their innovation is successful in improving teaching quality and student learning: when their own data analysis does not support this.
The evidence for a successful innovation
There is much material in the paper on the nature of the innovation, and there is evidence about student responses to it. Here, I am only concerned with the failure of the paper to offer a logical chain of argument to support their knowledge claim that the teaching innovation improved student achievement.
There are (to my reading – please judge for yourself if you can access the paper) some slight ambiguities in some parts of the description of the collection and analysis of achievement data (see note 5 below), but the key indicator relied on by Li, Ouyang, Xu and Zhang is the average score achieved by students in four teaching groups, three of which experienced the teaching innovation (these are denoted collectively as the 'the experimental group') and one group which did not (denoted as 'the control group', although there is no control of variables in the study 1). Each class comprised of 40 students.
The study is not published open access, so I cannot reproduce the copyright figures from the paper here, but below I have drawn a graph of these key data:
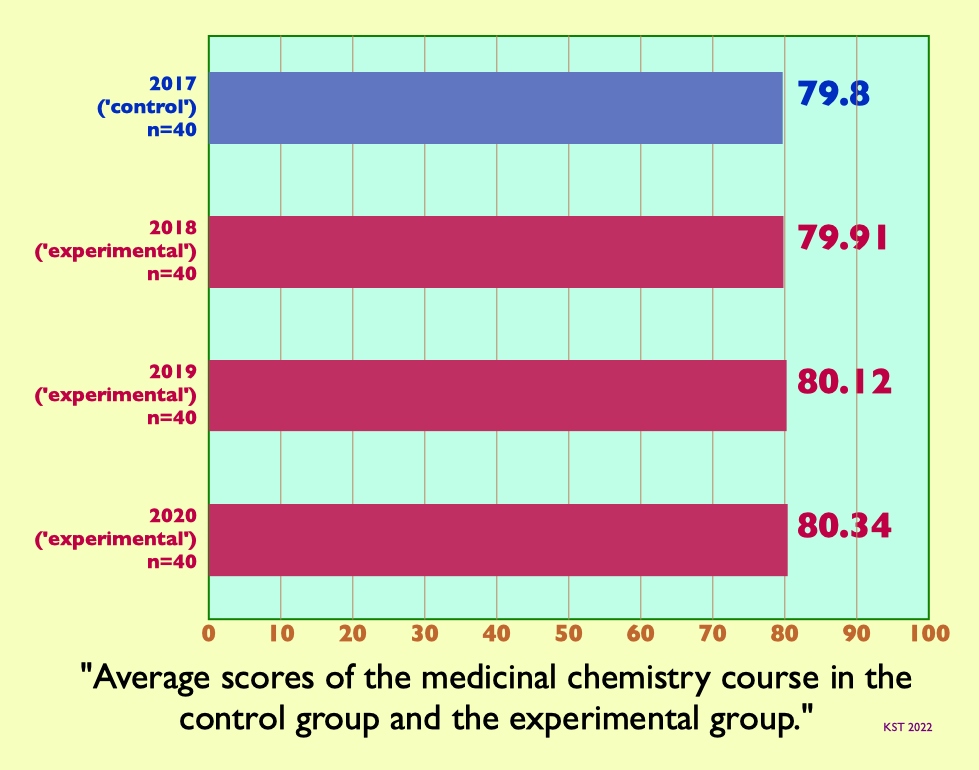
It is on the basis of this set of results that Li and colleagues claim that "the average score showed a constant upward trend, and a steady increase was found". Surely, anyone interrogating these data might have pause to wonder if that is the most authentic description of the pattern of scores year on year.
Does anyone teaching in a university really think that assessment methods are good enough to produce average class scores that are meaningful to 3 or 4 significant figures. To a more reasonable level of precision, nearest %age point (which is presumably what these numbers are – that is not made explicit), the results were:
Cohort | Average class score |
2017 | 80 |
2018 | 80 |
2019 | 80 |
2020 | 80 |
When presented to a realistic level of precision, the obvious pattern is…no substantive change year on year!
A truncated graph
In their paper, Li and colleagues do present a graph to compare the average results in 2017 with (not 2018, but) 2019 and 2020, somewhat similar to the one I have reproduced here which should have made it very clear how little the scores varied between cohorts. However, Li and colleagues did not include on their axis the full range of possible scores, but rather only included a small portion of the full range – from 79.4 to 80.4.
This is a perfectly valid procedure often used in science, and it is quite explicitly done (the x-axis is clearly marked), but it does give a visual impression of a large spread of scores which could be quite misleading. In effect, their Figure 4b includes just a slither of my graph above, as shown below. If one takes the portion of the image below that is not greyed out, and stretches it to cover the full extent of the x axis of a graph, that is what is presented in the published account.
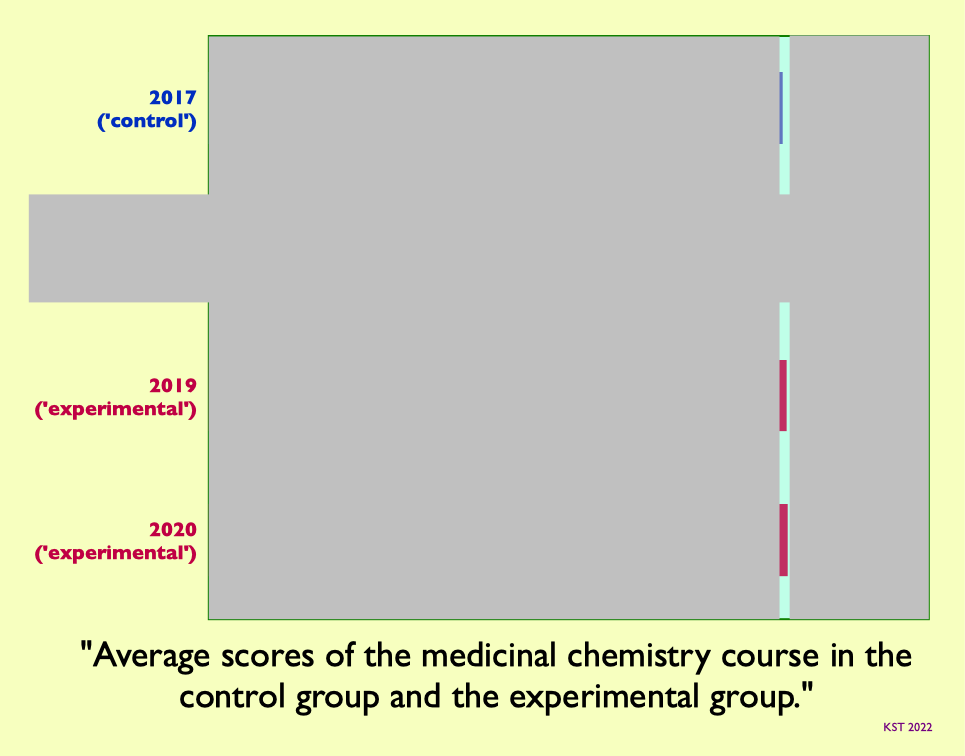

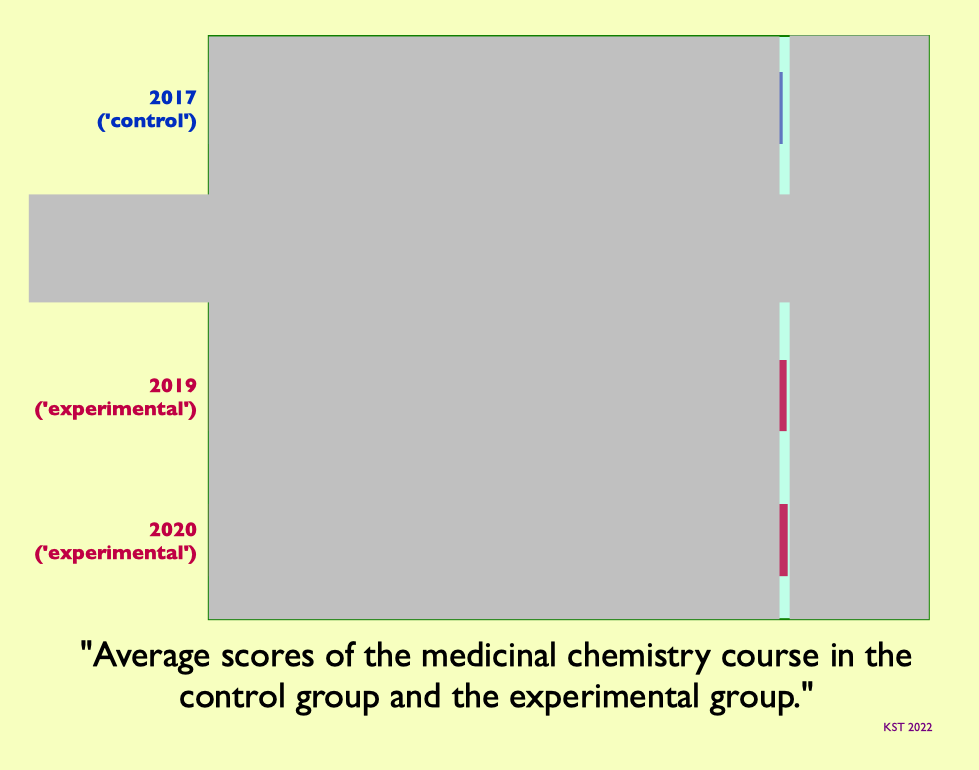
What might have caused those small variations?
If anyone does think that differences of a few tenths of a percent in average class scores are notable, and that this demonstrates increasing student achievement, then we might ask what causes this?
Li and colleagues seem to be convinced that the change in teaching approach caused the (very modest) increase in scores year on year. That would be possible. (Indeed, Li et al seem to be arguing that the very, very modest shift from 2017 to subsequent years was due to the change of teaching approach; but the not-quite-so-modest shifts from 2018 to 2019 to 2020 are due to developing teacher competence!) However, drawing that conclusion requires making a ceteris paribus assumption: that all other things are equal. That is, that any other relevant variables have been controlled.
Read about confounding variables
Another possibility however is simply that each year the teaching team are more familiar with the science, and have had more experience teaching it to groups at this level. That is quite reasonable and could explain why there might be a modest increase in student outcomes on a course year on year.
Non-equivalent groups of students?
However, a big assumption here is that each of the year groups can be considered to be intrinsically the same at the start of the course (and to have equivalent relevant experiences outside the focal course during the programme). Often in quasi-experimental studies (where randomisation to conditions is not possible 1) a pre-test is used to check for equivalence prior to the innovation: after all, if students are starting from different levels of background knowledge and understanding then they are likely to score differently at the end of a course – and no further explanation of any measured differences in course achievement need be sought.
Read about testing for initial equivalence
In experiments, you randomly assign the units of analysis (e.g., students) to the conditions, which gives some basis for at least comparing any differences in outcomes with the variations likely by chance. But this was not a true experiment as there was no randomisation – the comparisons are between successive year groups.
In Li and colleagues' study, the 40 students taking the class in 2017 are implicitly assumed equivalent to the 40 students taking the class in each of the years 20818-2020: but no evidence is presented to support this assumption. 3
Yet anyone who has taught the same course over a period of time knows that even when a course is unchanged and the entrance requirements stable, there are naturally variations from one year to the next. That is one of the challenges of educational research (Taber, 2019): you never can "take two identical students…two identical classes…two identical teachers…two identical institutions".
Novelty or expectation effects?
We would also have to ignore any difference introduced by the general effect of there being an innovation beyond the nature of the specific innovation (Taber, 2019). That is, students might be more attentive and motivated simply because this course does things differently to their other current courses and past courses. (Perhaps not, but it cannot be ruled out.)
The researchers are likely enthusiastic for, and had high expectations for, the innovation (so high that it seems to have biased their interpretation of the data and blinded them to the obvious problems with their argument) and much research shows that high expectation, in its own right, often influences outcomes.
Read about expectancy effects in studies
Equivalent examination questions and marking?
We also have to assume the assessment was entirely equivalent across the four years. 4 The scores were based on aggregating a number of components:
"The course score was calculated on a percentage basis: attendance (5%), preclass preview (10%), in-class group presentation (10%), postclass mind map (5%), unit tests (10%), midterm examination (20%), and final examination (40%)."
Li, et al, 2022, p.1858
This raises questions about the marking and the examinations:
- Are the same test and examination questions used each year (that is not usually the case as students can acquire copies of past papers)?
- If not, how were these instruments standardised to ensure they were not more difficult in some years than others?
- How reliable is the marking? (Reliable meaning the same scores/mark would be assigned to the same work on a different occasion.)
These various issues do not appear to have been considered.
Change of assessment methodology?
The description above of how the students' course scores were calculated raises another problem. The 2017 cohort were taught by "direct instruction". This is not explained as the authors presumably think we all know exactly what that is : I imagine lectures. By comparison, in the innovation (2018-2020 cohorts):
"The preclass stage of the SCTBL strategy is the distribution of the group preview task; each student in the group is responsible for a task point. The completion of the preview task stimulates students' learning motivation. The in-class stage is a team presentation (typically PowerPoint (PPT)), which promotes students' understanding of knowledge points. The postclass stage is the assignment of team homework and consolidation of knowledge points using a mind map. Mind maps allow an orderly sorting and summarization of the knowledge gathered in the class; they are conducive to connecting knowledge systems and play an important role in consolidating class knowledge."
Li, et al, 2022, p.1856, emphasis added.
Now the assessment of the preview tasks, the in-class group presentations, and the mind maps all contributed to the overall student scores (10%, 10%, 5% respectively). But these are parts of the innovative teaching strategy – they are (presumably) not part of 'direct instruction'. So, the description of how the student class scores were derived only applies to 2018-2020, and the methodology used in 2017 must have been different. (This is not discussed in the paper.) 5
A quarter of the score for the 'experimental' groups came from assessment components that could not have been part of the assessment regime applied to the 2017 cohort. At the very least, the tests and examinations must have been more heavily weighed into the 'control' group students' overall scores. This makes it very unlikely the scores can be meaningfully directly compared from 2017 to subsequent years: if the authors think otherwise they should have presented persuasive evidence of equivalence.
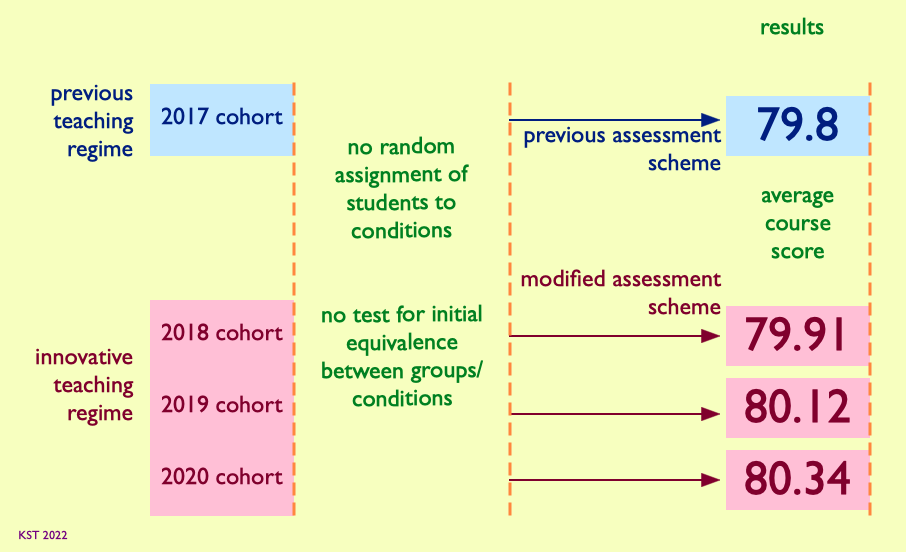
So, groups that we cannot assume are equivalent are assessed in ways that we cannot assume to be equivalent and obtain nearly identical average levels of achievement. Despite that, Li and colleagues want to persuade us that the very modest differences in average scores between the 'control' and 'experimental' groups (which is actually larger between different 'experimental group' cohorts than between the 'control' group and the successive 'experimental' cohort) are large enough to be significant and demonstrate their teaching innovation improves student achievement.
Statistical inference
So, even if we thought shifts of less than a 1% average in class achievement were telling, there are no good reasons to assume they are down to the innovation rather than some other factor. But Li and colleagues use statistical tests to tell them whether differences between the 'control' and 'experimental' conditions are significant. They find – just what anyone looking at the graph above would expect – "there is no significant difference in average score" (p.1860).
The scientific convention in using such tests is that the choice of test, and confidence level (e.g., a probability of p<0.05 to be taken as significant) is determined in advance, and the researchers accept the outcomes of the analysis. There is a kind of contract involved – a decision to use a statistical test (chosen in advance as being a valid way of deciding the outcome of an experiment) is seen as a commitment to accept its outcomes. 2 This is a form of honesty in scientific work. Just as it is not acceptable to fabricate data, nor is is acceptable to ignore experimental outcomes when drawing conclusions from research.
Special pleading is allowed in mitigation (e.g., "although our results were non-significant, we think this was due to the small samples sizes, and suggest that further research should be undertaken with large groups {and we are happy to do this if someone gives us a grant}"), but the scientist is not allowed to simply set aside the results of the analysis.
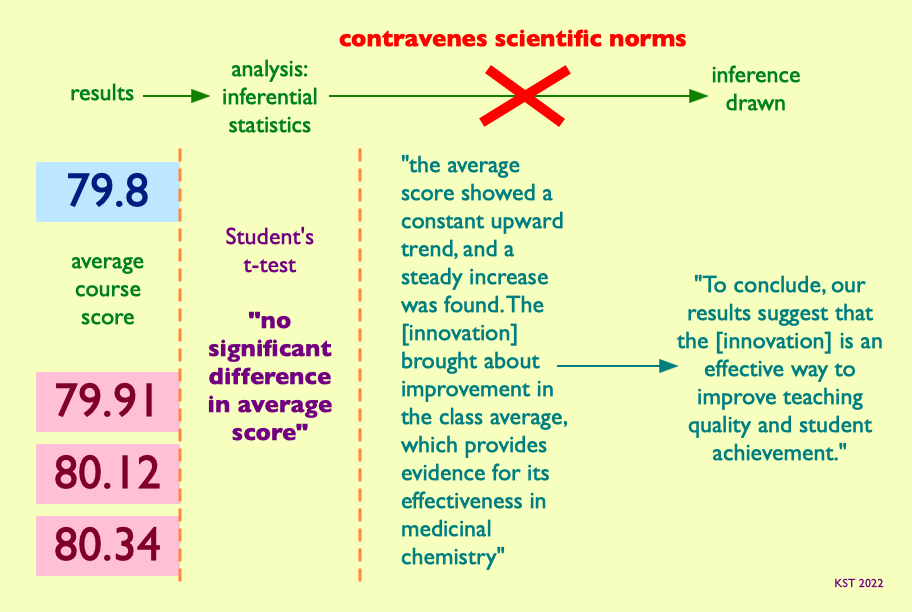
Yet setting aside the results of their analysis is what Li and colleagues do. They carry out an analysis, then simply ignore the findings, and conclude the opposite:
"To conclude, our results suggest that the SCTBL method is an effective way to improve teaching quality and student achievement."
Li, et al, 2022, p.1861
It was this complete disregard of scientific values, rather than the more common failure to appreciate that they were not comparing like with like, that I found really shocking – and led to me writing a formal letter to the journal. Not so much surprise that researchers might do this (I know how intoxicating research can be, and how easy it is to become convinced in one's ideas) but that the peer reviewers for the Journal of Chemical Education did not make the firmest recommendation to the editor that this manuscript could NOT be published until it was corrected so that the conclusion was consistent with the findings.
This seems a very stark failure of peer review, and allows a paper to appear in the literature that presents a conclusion totally unsupported by the evidence available and the analysis undertaken. This also means that Li, Ouyang, Xu and Zhang now have a publication on their academic records that any careful reader can see is critically flawed – something that could have been avoided had peer reviewers:
- used their common sense to appreciate that variations in class average scores from year to year between 79.8 and 80.3 could not possibly be seen as sufficient to indicate a difference in the effectiveness of teaching approaches;
- recommended that the authors follow the usual scientific norms and adopt the reasonable scholarly value position that the conclusion of your research should follow from, and not contradict, the results of your data analysis.
Work cited:
- Li, W., Ouyang, Y., Xu, J., & Zhang, P. (2022). Implementation of the Student-Centered Team-Based Learning Teaching Method in a Medicinal Chemistry Curriculum. Journal of Chemical Education, 99(5), 1855-1862. https://doi.org/10.1021/acs.jchemed.1c00978
- Taber, K. S. (2013). Classroom-based Research and Evidence-based Practice: An introduction (2nd ed.). London: Sage.
- Taber, K. S. (2019). Experimental research into teaching innovations: responding to methodological and ethical challenges. Studies in Science Education, 55(1), 69-119. doi:10.1080/03057267.2019.1658058 [Download manuscript version]
Notes
1 Strictly the 2017 cohort has the role of a comparison group, but NOT a control group as there was no randomisation or control of variables, so this was not a true experiment (but a 'quasi-experiment'). However, for clarity, I am here using the original authors' term 'control group'.
Read about experimental research design
2 Some journals are now asking researchers to submit their research designs and protocols to peer review BEFORE starting the research. This prevents wasted effort on work that is flawed in design. Journals will publish a report of the research carried out according to an accepted design – as long as the researchers have kept to their research plans (or only made changes deemed necessary and acceptable by the journal). This prevents researchers seeking to change features of the research because it is not giving the expected findings and means that negative results as well as positive results do get published.
3 'Implicitly' assumed as nowhere do the authors state that they think the classes all start as equivalent – but if they do not assume this then their argument has no logic.
Without this assumption, their argument is like claiming that growing conditions for tree development are better at the front of a house than at the back because on average the trees at the front are taller – even though fast-growing mature trees were planted at the front and slow-growing saplings at the back.
4 From my days working with new teachers, a common rookie mistake was assuming that one could tell a teaching innovation was successful because students achieved an average score of 63% on the (say, acids) module taught by the new method when the same class only averaged 46% on the previous (say, electromagnetism) module. Graduate scientists would look at me with genuine surprise when I asked how they knew the two tests were of comparable difficulty!
Read about why natural scientists tend to make poor social scientists
5 In my (rejected) letter to the Journal of Chemical Education I acknowledged some ambiguity in the paper's discussion of the results. Li and colleagues write:
"The average scores of undergraduates majoring in pharmaceutical engineering in the control group and the experimental group were calculated, and the results are shown in Figure 4b. Statistical significance testing was conducted on the exam scores year to year. The average score for the pharmaceutical engineering class was 79.8 points in 2017 (control group). When SCTBL was implemented for the first time in 2018, there was a slight improvement in the average score (i.e., an increase of 0.11 points, not shown in Figure 4b). However, by 2019 and 2020, the average score increased by 0.32 points and 0.54 points, respectively, with an obvious improvement trend. We used a t test to test whether the SCTBL method can create any significant difference in grades among control groups and the experimental group. The calculation results are shown as follows: t1 = 0.0663, t2 = 0.1930, t3 =0.3279 (t1 <t2 <t3 <t𝛼, t𝛼 =2.024, p>0.05), indicating that there is no significant difference in average score. After three years of continuous implementation of SCTBL, the average score showed a constant upward trend, and a steady increase was found. The SCTBL method brought about improvement in the class average, which provides evidence for its effectiveness in medicinal chemistry."
Li, et al, 2022, p.1858-1860, emphasis added
This appears to refer to three distinct measures:
- average scores (produced by weighed summations of various assessment components as discussed above)
- exam scores (perhaps just the "midterm examination…and final examination", or perhaps just the final examination?)
- grades
Formal grades are not discussed in the paper (the word is only used in this one place), although the authors do refer to categorising students into descriptive classes ('levels') according to scores on 'assessments', and may see these as grades:
"Assessments have been divided into five levels: disqualified (below 60), qualified (60-69), medium (70-79), good (80-89), and excellent (90 and above)."
Li, et al, 2022, p.1856, emphasis added
In the longer extract above, the reference to testing difference in "grades" is followed by reporting the outcome of the test for "average score":
"We used a t test to test …grades …The calculation results … there is no significant difference in average score"
As Student's t-test was used, it seems unlikely that the assignment of students to grades could have been tested. That would surely have needed something like the Chi-squared statistic to test categorical data – looking for an association between (i) the distributions of the number of students in the different cells 'disqualified', 'qualified', 'medium', 'good' and 'excellent'; and (ii) treatment group.
Presumably, then, the statistical testing was applied to the average course scores shown in the graph above. This also makes sense because the classification into descriptive classes loses some of the detail in the data and there is no obvious reason why the researchers would deliberately chose to test 'reduced' data rather than the full data set with the greatest resolution.